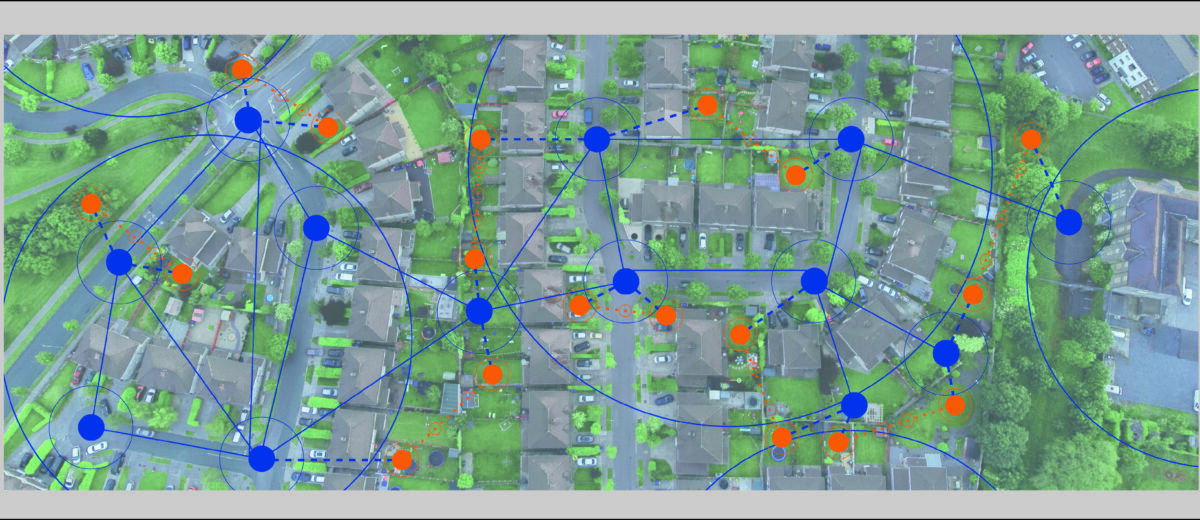
How Sewall’s Newest Team Member is Advancing Human-Centric Geospatial Tech
By Caroline Engel
At Sewall, we don’t just adopt the latest in geospatial innovation—we help build it. And we’re proud to introduce the newest member of our team who’s doing exactly that: Brendan Hall. Brendan brings deep technical skill combined with research that’s poised to reshape how geospatial systems interpret and respond to human-centered questions about space and place.
Through his recently published work on Conceptual Neighborhood Graphs (CNGs) in raster (pixel-based) data, Brendan is introducing a new level of formally defined CNGs to GIS—one that reflects how humans naturally understand and describe spatial relationships.
The Problem: GIS Doesn’t Think Like We Do
An example by Brendan Hall: let’s say you’re analyzing satellite imagery and tracking a deer’s movement. Traditional GIS might log its location in “Position 1” before jumping a fence, and in “Position 2” after it jumps a fence—but misses the fact that it intersected the fence in between. Humans would understand this intuitively. GIS systems today? Not so much. They treat spatial relationships as binary and static—either true or false—without capturing the in-between or neighboring states that may exist beyond those identified by satellite imagery. By contrast, intelligent systems can use CNGs to infer and understand additional likely spatial configurations.
What Are Conceptual Neighborhood Graphs (CNGs)?
CNGs are a way of modeling how spatial relationships change over time and space—using terms we actually use in conversation: “near,” “inside,” “along,” or “just past.” These spatial prepositions reflect our natural cognitive ability to understand space, and CNGs mirror this.
Brendan’s research formalized the first full CNG models for raster data—which is the dominant form in satellite imagery and remote sensing. This means that for the first time, computers can start to track how one region relates to another—and model those changing relationships using a conceptual neighborhood graph.
Integrating CNGs into GIS opens the door to smarter, more intent-aware spatial systems. These tools could rank query results based on relatedness, fill in gaps in temporal data, and offer a more expressive interface for both experts and non-specialists. By using neighborhood graphs, we enable the system to provide the next best results to a user’s query. For example, a system powered by CNGs could distinguish between “a tree near the yard” and “a tree just inside the yard,” giving planners, analysts, and decision-makers richer context.
Brendan explains that this kind of reasoning aligns with how we already think and talk about space—even before we develop language. It’s built into the human condition. Concepts like “next to” or “inside” are universally understood across cultures, and his work is creating the bridge between that intuitive reasoning and machine understanding.
From urban planning to disaster response, from agriculture to AI-based navigation, this research has enormous implications. Systems using CNGs can better interpret qualitative queries (e.g., “a quiet place outside the city”) and provide ranked, context-aware answers instead of flat, binary results.
In Brendan’s words: “GIS tools have been working just fine, but they’ve been missing something. CNGs help us show users what they didn’t know they were missing.”
At Sewall, We’re Leaning Into the Future
Brendan’s work supports Sewall’s mission to deliver best-in-class geospatial software and solutions. By enabling smarter, more intuitive systems, we’re helping clients make decisions based not just on data—but on understanding. We at Sewall are excited to begin further integration of “CNGs research [which] will enable our clients to make better decisions based on the data we generate using these tools. I like the fact that it’s built into the human condition at a foundational level. It is something that will easily benefit not just GIS, but intelligence systems through its application.”—Brendan Hall
Want to Learn More?
Brendan recommends starting with a foundational piece that inspired much of his research: Naive Geography by Max J. Egenhofer and David M. Mark (1995). It introduces the idea that humans have an innate, language-independent way of reasoning about spatial relationships—one that CNGs are finally making machine-readable.
Read Naive Geography by Egenhofer & Mark (1995) https://www.researchgate.net/publication/2893373_Naive_Geography
Learn from the Research Itself
To read the publication and view Brendan Hall’s research directly, visit this link: https://www.mdpi.com/2220-9964/14/4/150
Acknowledgments
This work builds on foundational contributions by Max Egenhofer and was developed in close collaboration with co-author Matthew Dube, whose expertise was key to adapting and validating these spatial relationship models for use with raster (grid-based) data.
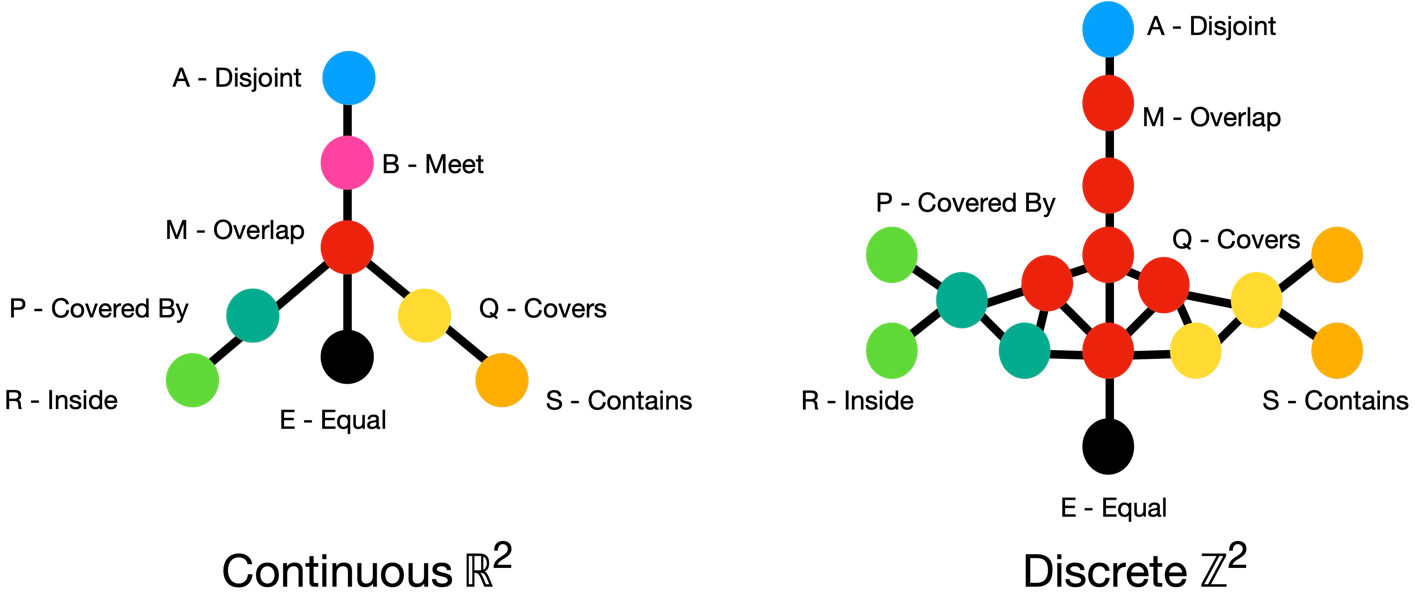
Continuous vs. Discrete Conceptual Neighborhoods
The continuous neighborhood (ℝ²) shows a simplified relational structure, while the discrete raster-based neighborhood (ℤ²) reveals additional states that arise from thick boundary regions not available in continuous space. These richer distinctions enable more nuanced spatial reasoning and improved inference in intelligent systems.
What This Image Shows: The image above compares how spatial relationships work in two types of spaces: continuous (left) and discrete (right). On the left, the continuous model (think smooth shapes like circles) shows simpler relationships, like “overlaps,” “inside,” or “disjoint.” On the right, the discrete model (think pixelated or grid-based data, like digital maps or satellite imagery) reveals a more detailed set of relationships. Because cells can “intersect” in more complex ways, new possibilities emerge—like partial overlaps generated by boundary regions which include a thickness, unlike their continuous counterparts. These extra
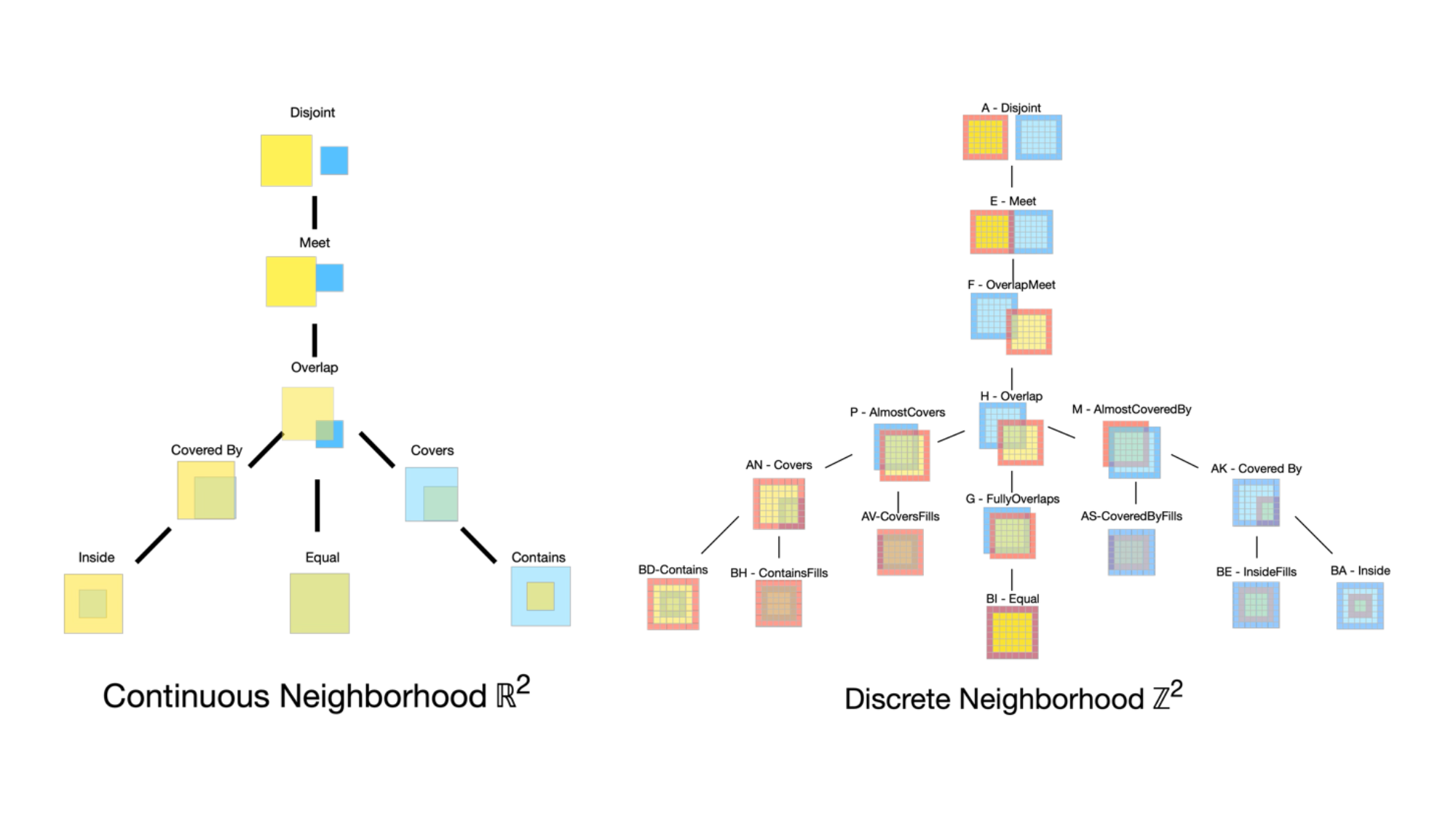